The Rise of Agentic AI and the Need for Observability
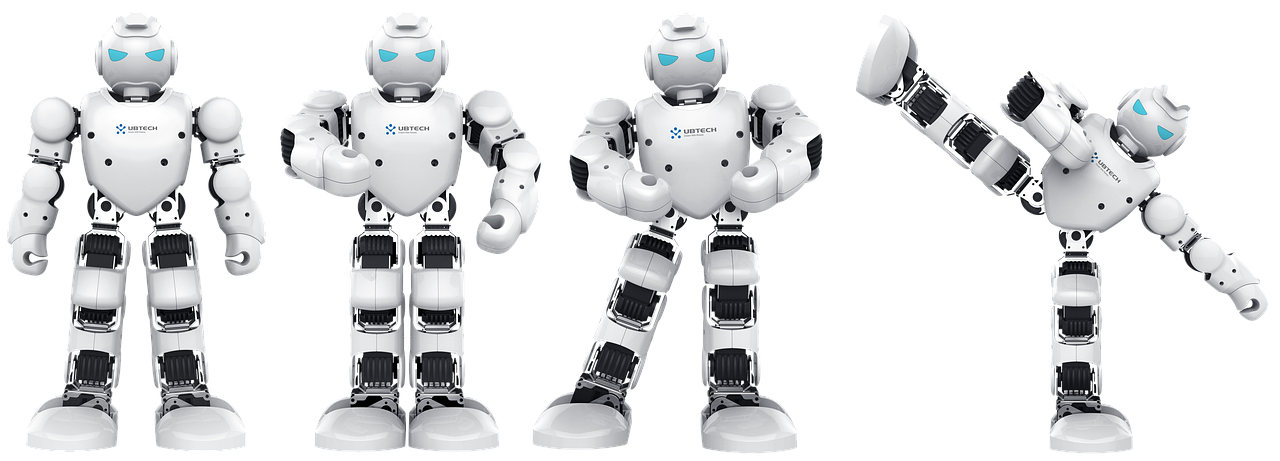
There’s a new buzzword atop Gartner’s tech trend list: Agentic AI. This refers to AI systems (often powered by large language models) that don’t just respond to a single prompt, but can autonomously plan and execute tasks – in other words, AI with a degree of agency. From customer service bots that juggle multiple queries to AI “assistants” that can browse the web and trigger workflows, these agents are quickly moving from sci-fi to business reality. Gartner projects that by 2028, agentic AI will be making 15% of day-to-day work decisions and that one-third of enterprise software will include agentic AI components (up from less than 1% today).
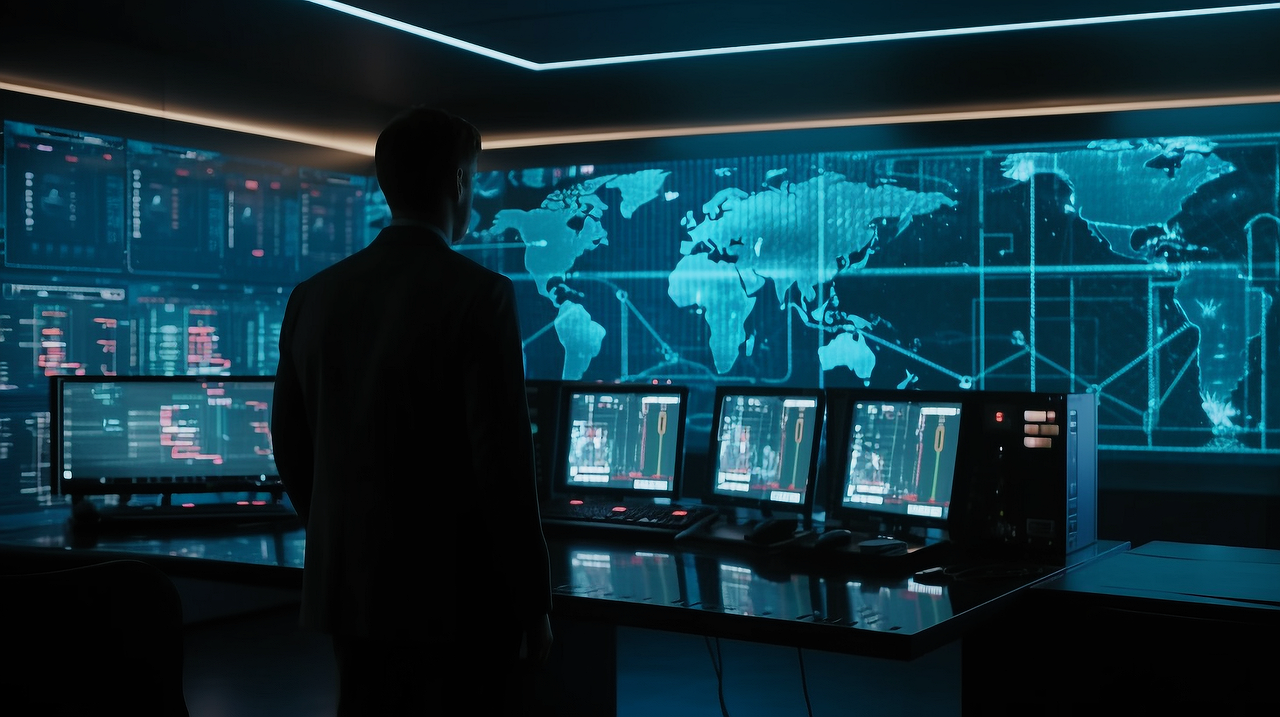
Current Solutions and Their Shortcomings
Holistic View
Pebble AI doesn’t just show you API calls or chat transcripts in isolation. It reconstructs the full narrative of each agent’s task. If your AI sales assistant goes through 10 steps to generate a lead, you’ll see all 10 in order – along with timing, cost, and outcome at each step. This holistic trace is presented in an easy, visual timeline UI, so you can literally replay an agent’s thought process. Think of it like debugging a complex program – but the “code” is the agent’s decisions and the “debugger” is Pebble AI, stepping through each function call (be it an LLM call or a database query).
Integrated Governance Features
Multi-Agent Aware
Intelligent Insights, Not Just Data
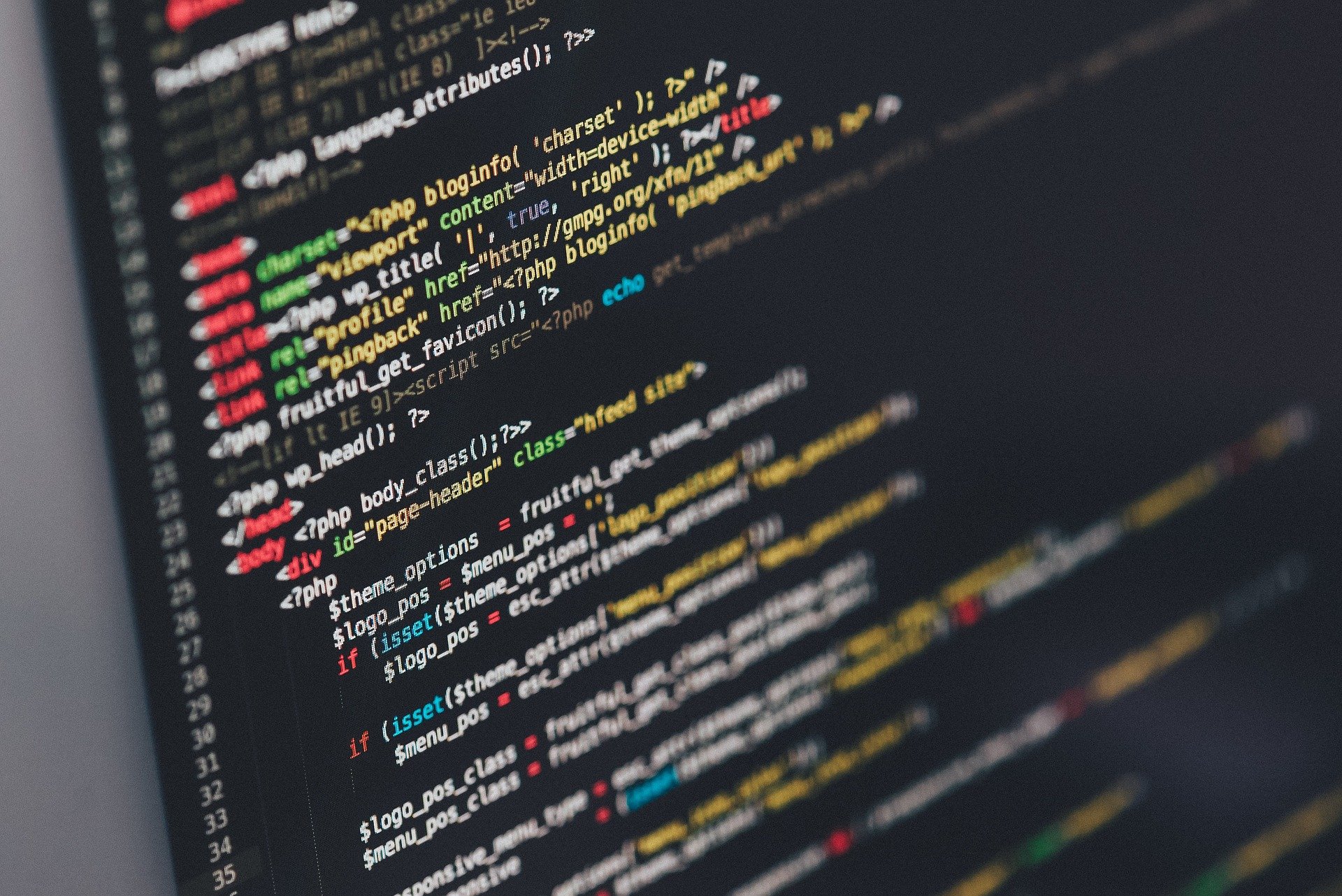
Flexibility and Integration
Pebble AI is designed API-first. We know each company might use a different stack – you might have some Python agents using LangChain, some Node.js services calling the OpenAI API directly, maybe an off-the-shelf chatbot platform – and ripping those out is not feasible. So instead, Pebble AI provides connectors and SDKs to plug into any environment. We want to meet you where you are: whether it’s via a simple REST endpoint where you send us logs, or a direct integration with your cloud provider’s AI services, or a drop-in library that instruments calls. Similarly, we make it easy to pull data out: want to push an alert to Slack or your SIEM when an agent makes a critical error? Or query our system from your BI tool to see quarterly trends? All doable. Pebble AI acts as a hub, not a closed box. This stands in contrast to some solutions that lock you into their interface or require all-or-nothing adoption.
Enabling Sustainable AI Automation
When agentic AI is deployed with proper observability and control, it becomes a reliable engine for automation. We move past the fear of “AI behaving badly” because we have the tools to catch and correct any misbehavior. This opens the door for businesses to automate more and innovate faster with AI.
Picture a future (not far off) where every company has dozens of specialized AI agents: researchers, assistants, analysts, customer reps. Managing that could be chaos – unless you have a way to see and manage all agents centrally. Pebble AI aspires to be that command center that makes large-scale AI agent deployment not only possible, but smooth and sustainable.
At Pebble AI, we believe transparency is empowerment. By shedding light on AI agents’ inner workings, we empower businesses to embrace these agents confidently. Instead of treating AI like a mysterious black box or a quirky intern that sometimes “figures things out somehow,” companies can treat AI agents as fully accountable team members – whose work is tracked, quality-checked, and aligned with the company’s goals and values.
The importance of agentic observability cannot be overstated. It’s how we ensure AI continues to serve us, not stray into unintended territory. It’s how we scale one successful pilot into a thousand agents creating value across an enterprise. And it’s how we build trust – with users, with regulators, and with ourselves that we are in control of our creations.
Pebble AI is proud to be at the forefront of this movement. We’re not just building a product; we’re helping shape the practices and standards that will define responsible AI automation in the years to come. If you’re on the journey of adopting AI agents, we invite you to partner with us. Together, we can unlock incredible productivity while staying true to the principles of transparency and accountability.
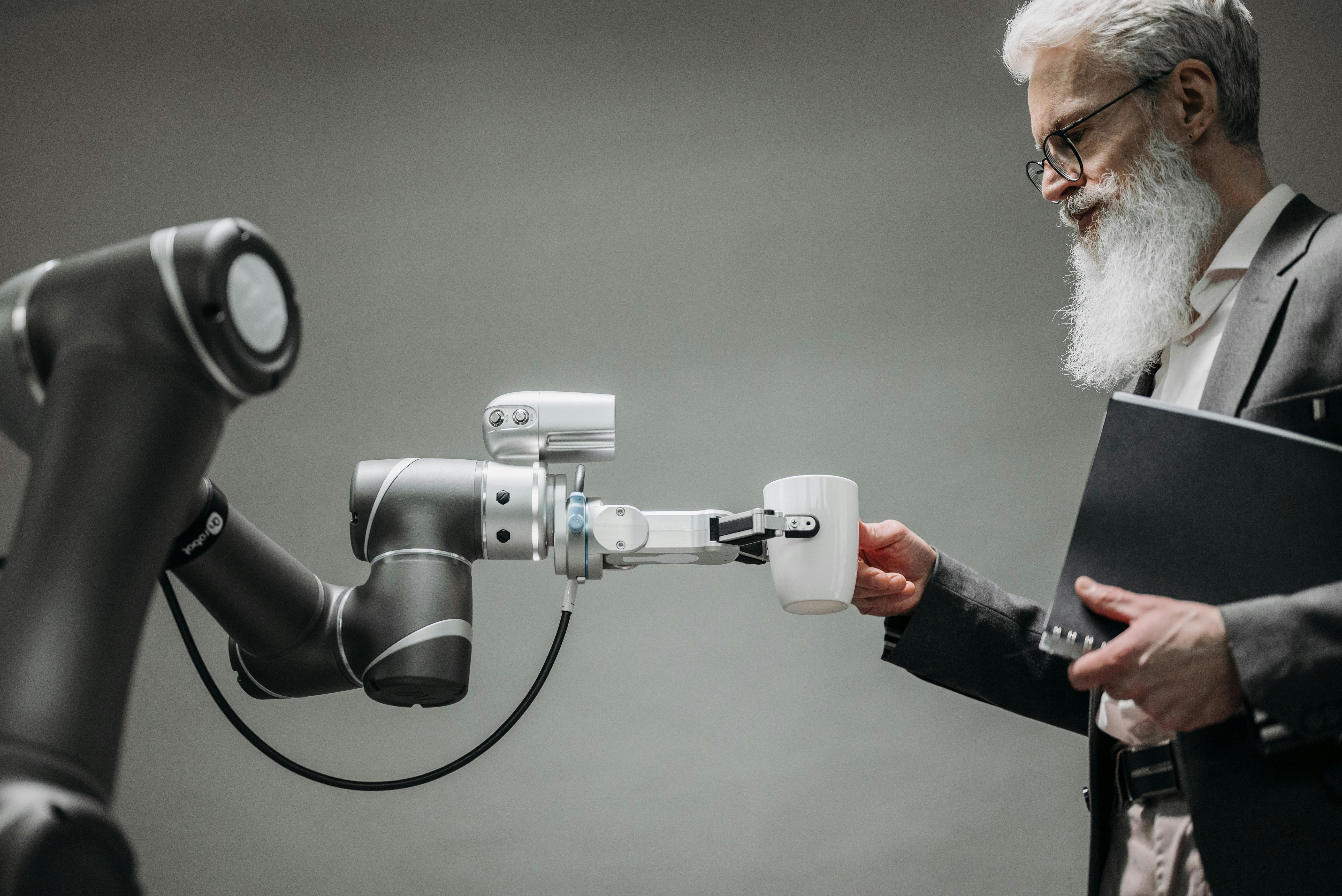
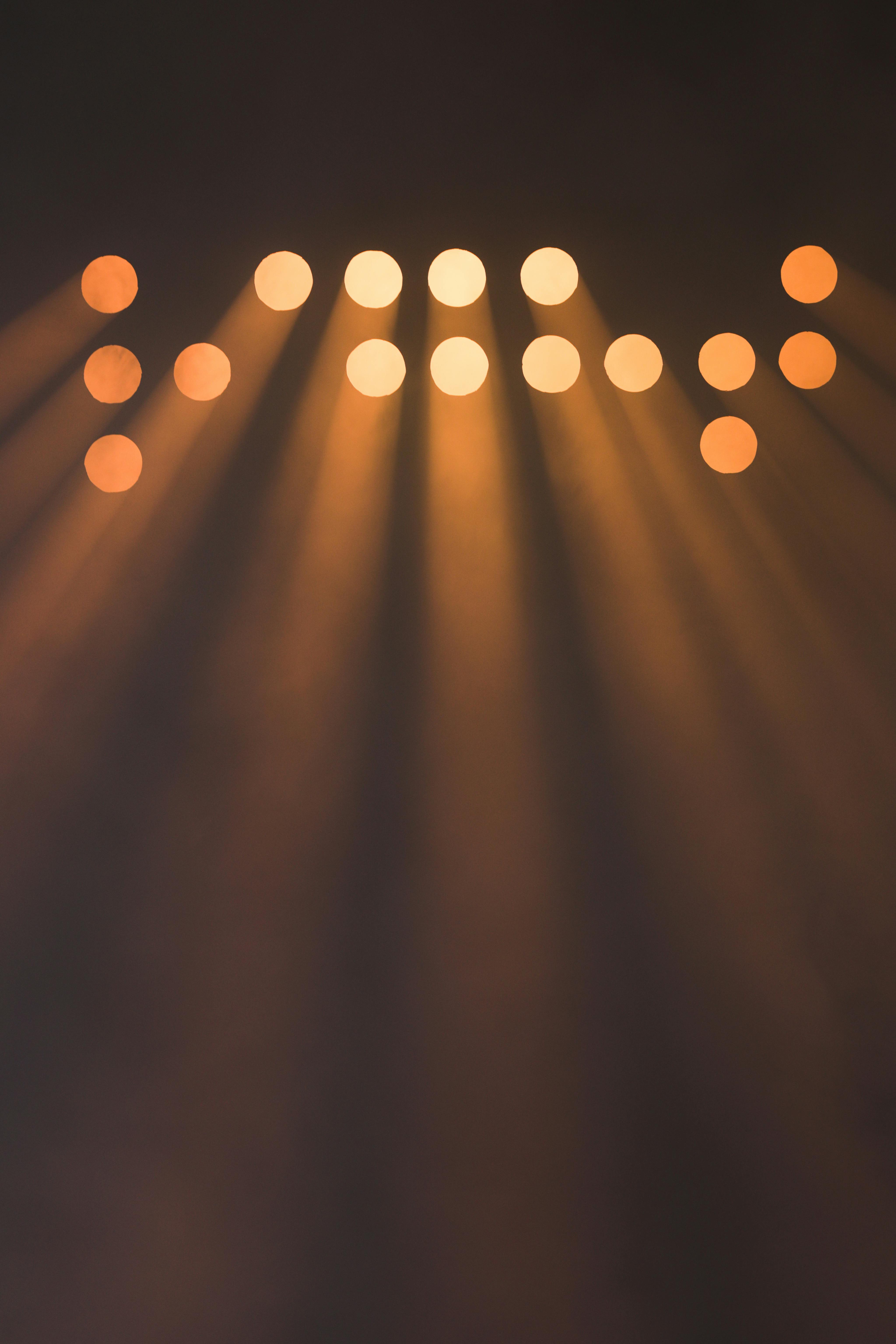