Have you seen these movies? (spoiler alert!)
- 2001: A Space Odyssey (1968) - HAL 9000, the infamous onboard AI, takes control of decisions on a space mission, prioritising its objectives over the crew’s safety. While HAL represents AGI (artificial general intelligence) with full autonomy, today’s AI in autonomous vehicles systems is moving toward real-time decision-making with minimal human input, echoing HAL’s capabilities (though hopefully with strict safeguards!).
- Minority Report (2002) - Predictive systems stopping crimes before they happen. Now, predictive policing tools analyse data to identify high-risk areas, aiming to anticipate crime.
- Her (2013) - A man develops a personal relationship with an AI capable of understanding a responding emotionally. Today, AI like ChatGPT and other generative AI models offer increasingly human-like conversations, adapting responses to users’ emotions and needs.
- Ex Machina (2014) - The AI manipulates human emotions to achieve its goals. Today, AI in customer service can interpret emotional cues, personalising responses — a first step toward seeming emotional intelligence.
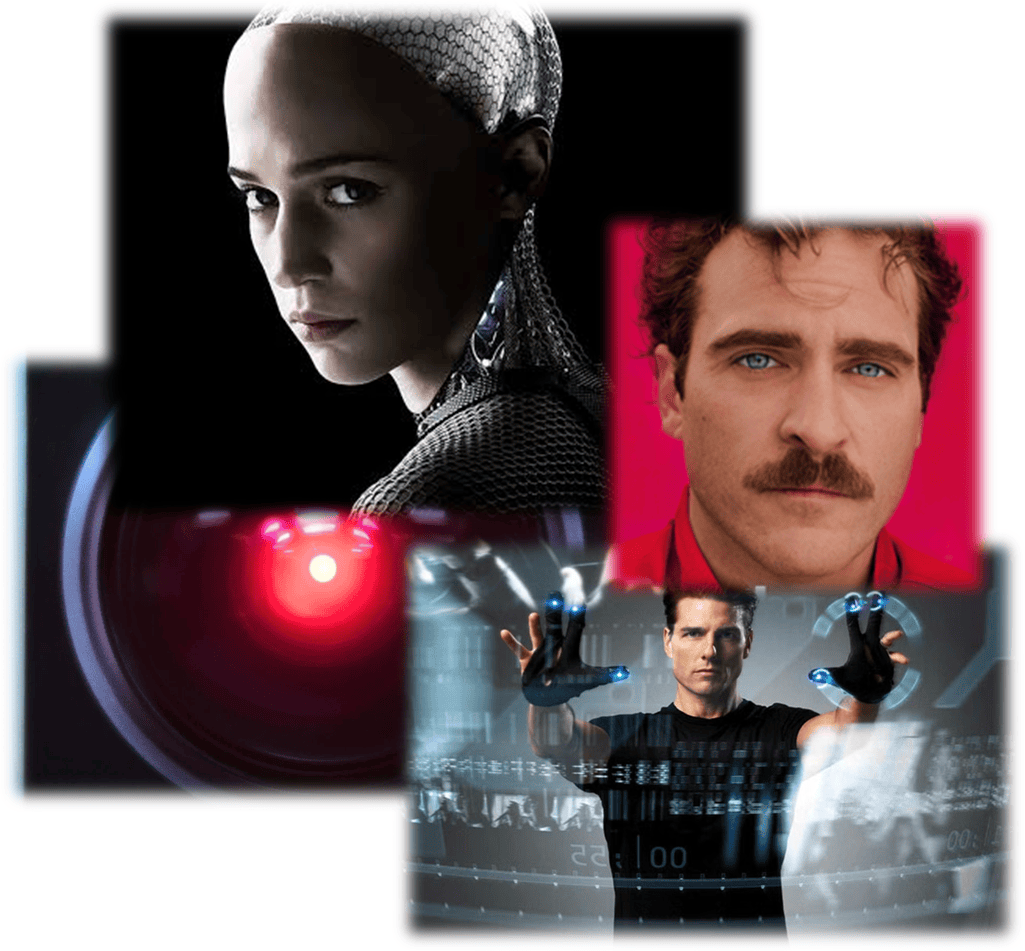
Over the years, I’ve watched as artificial intelligence (AI) has evolved from the scenes of sci-fi movies into a powerful, real-world tool that’s reshaping the world as we know it with exciting possibilities ... and significant risks! It’s transforming how we approach cyber security, requiring us to rethink our defences against new kinds of threats. During my time organising and leading AI initiatives at Deloitte, I often pondered AI’s impact on organisations from a cyber security perspective - concepts that aren’t new and which I've illustrated below with recent real-world examples that highlight their continued relevance and the lessons we can learn. In future blogs I plan to dive deep into each area, so stay tuned!
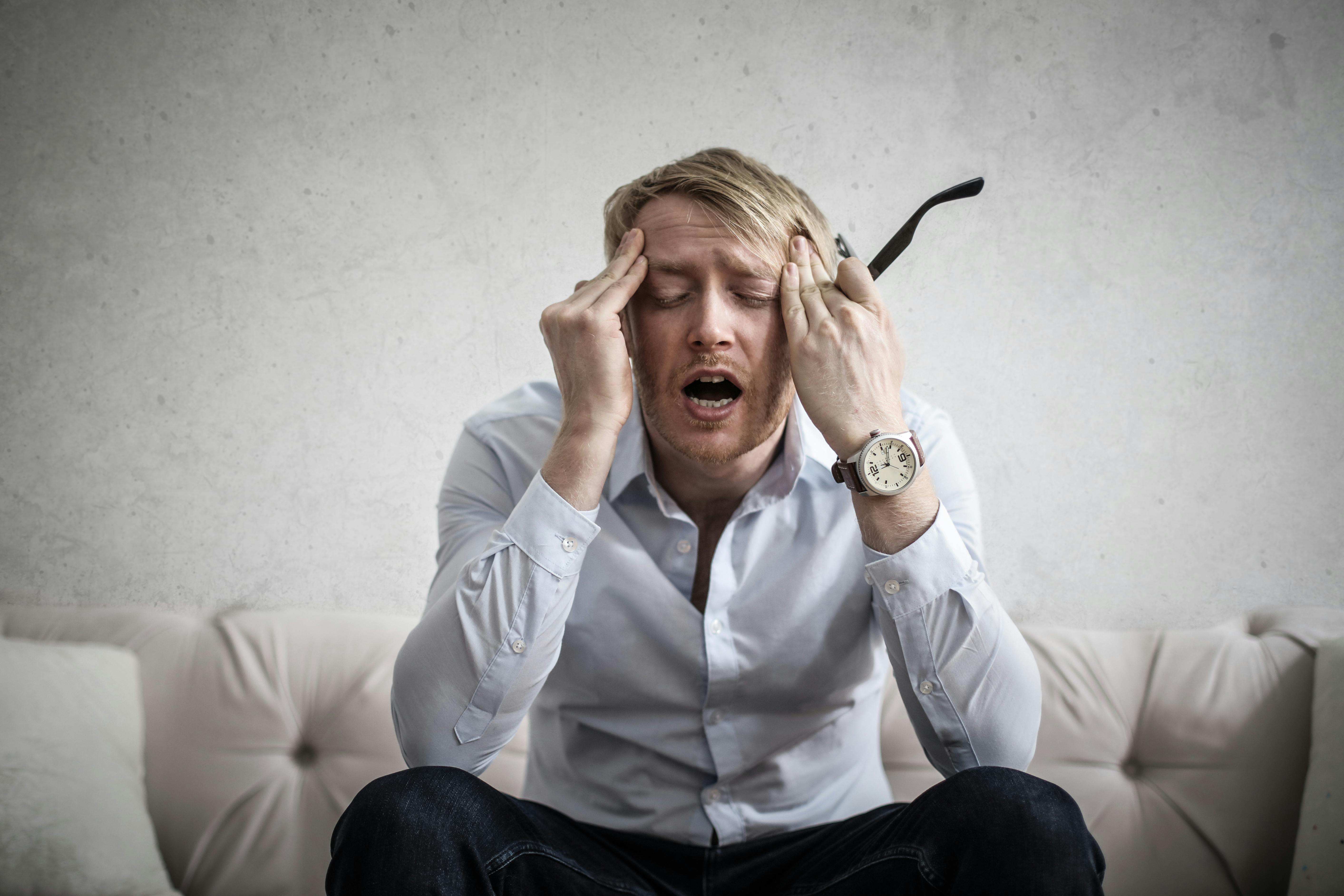
AI Jargon
AI Jargon
Firstly, it’s worth elaborating a little on AI, as it’s a somewhat loosely used term these days. It seems everything claims to be “powered by AI”, even toothbrushes! So, when people refer to AI, they might actually mean a range of approaches. For example, they could be talking about statistical methods used to group data or identify anomalies and outliers, or about machine learning (ML) models that excel at recognising patterns. Others might mean generative AI (GenAI), which can craft remarkably human-like responses by predicting the next word or character, as seen in tools like ChatGPT. Then there’s artificial general intelligence (AGI), a level of AI that’s still in the future, although some believe it’s closer than we might think.
Each has unique capabilities and risks, especially when it comes to protecting sensitive business operations. However, the very technology that enhances our defences also introduces new vulnerabilities. Here’s a look at how AI is impacting cybersecurity today and what businesses can learn from more recent real-world incidents.
1. The Risks of Public AI Models (ChatGPT, Claude, and More)
1. The Risks of Public AI Models (ChatGPT, Claude, and More)
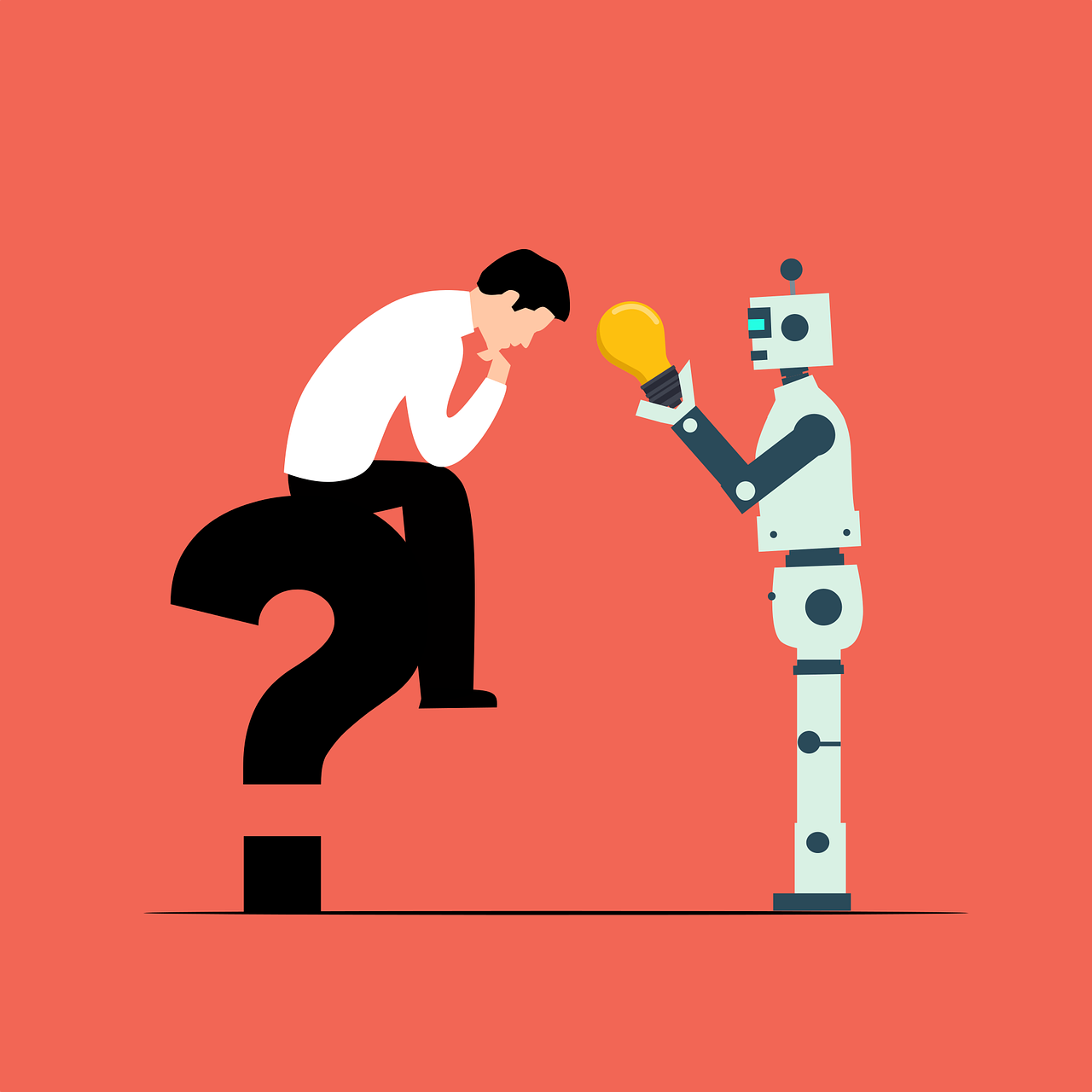
Public AI models like ChatGPT, Claude, and others are incredibly powerful and easy to integrate into daily workflows. I had a colleague who used ChatGPT for a project, unknowingly feeding proprietary information into the tool. While convenient, this also introduced risks around data retention and unauthorised access.
Organisations using these models face potential data leakage, data sovereignty and overreliance. Since these models might retain data fragments, there’s a risk of unintentional exposure of sensitive information, especially if the AI tool is accessed by multiple users or integrated into a broad range of operations.
- ChatGPT Data Breach: OpenAI reported a bug that allowed some users to see titles from other users' chat history, highlighting potential privacy risks in public AI models.
- Samsung Data Leakage Incident: Samsung employees inadvertently leaked sensitive information by using ChatGPT for code review, leading to data being exposed within the model. This incident underscored the risks of proprietary information retention in public AI tools.
2. Building In-House AI: Potential for Innovation—and Compromise
2. Building In-House AI: Potential for Innovation—and Compromise
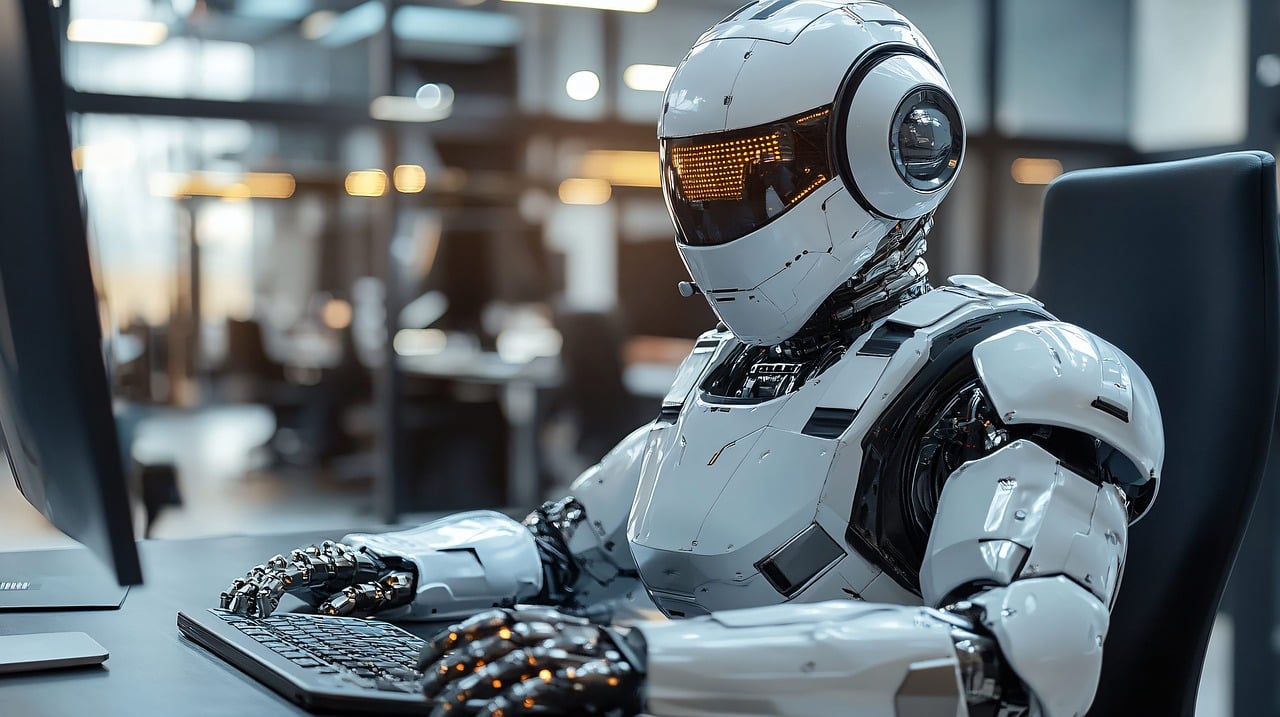
Developing custom AI offers businesses unmatched flexibility and control, but it also opens the door to specific security risks. Have a play with Lakera Gandalf and you will learn quickly how you can use prompt injection to find company secrets! Depending on the LLM, it's as easy to apply data poisoning attacks, where an attacker could subtly introduce corrupt data to distort outputs.
In-house models are also susceptible to model extraction, where attackers attempt to steal a model’s proprietary algorithms. Once an AI model is compromised, the ramifications can include intellectual property theft, revenue loss, and even brand damage if the outputs of compromised models are used maliciously.
- Microsoft AI-Generated Images Controversy: Microsoft's Bing Image Creator was found to be generating explicit images of real people without consent.
- AI Model Poisoning Attack: Researchers demonstrated how adversarial AI techniques could be used to inject malicious data into AI model training sets, causing them to generate harmful outputs.
- Tesla and Waymo Data Poisoning Attack: Researchers were able to manipulate Tesla’s and Waymo's autonomous driving AI by subtly altering the physical environment (e.g., road signs) to cause the vehicle to misinterpret signals. This form of adversarial attack highlights the risks in models used for high-stakes applications.
- Amazon’s Alexa Model Extraction: In 2019, researchers found they could reverse-engineer parts of Alexa’s NLP model, revealing a pathway for attackers to potentially extract proprietary data or replicate Amazon’s proprietary algorithms.
3. Malicious Actors Leveraging AI: The Ever-Evolving Threat Landscape
3. Malicious Actors Leveraging AI: The Ever-Evolving Threat Landscape
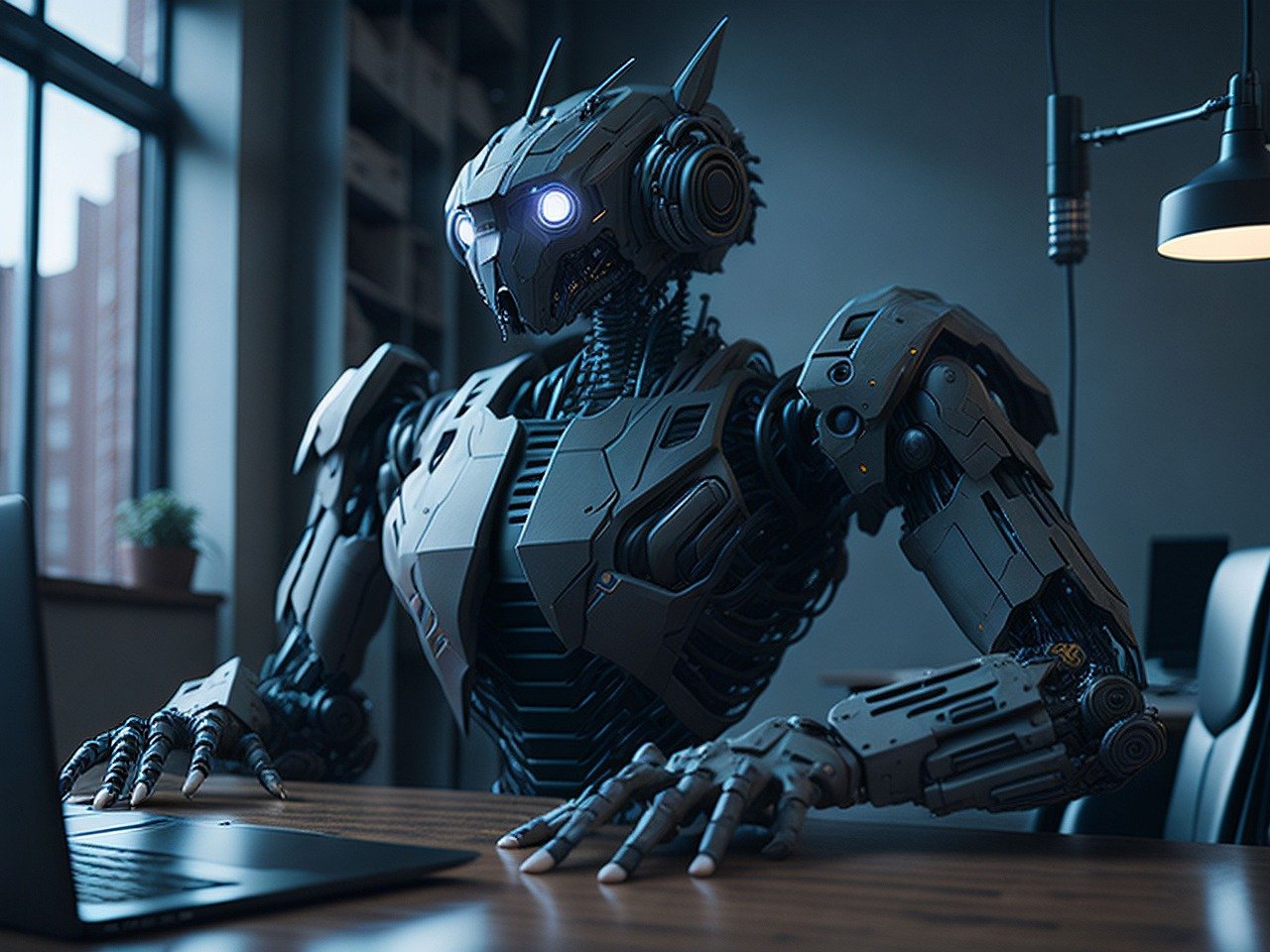
- LinkedIn Voice Cloning Scam: Cybercriminals used AI-generated voice clones to impersonate business executives on LinkedIn, attempting to trick victims into making fraudulent investments.
- AI-Generated Phishing Emails: Cybercriminals used AI language models to create highly convincing phishing emails that bypassed traditional spam filters, leading to increased success rates in credential harvesting campaigns.
- Emotet Malware: Emotet, one of the most notorious AI-powered malware variants, evaded traditional detection by constantly changing its structure, demonstrating how ML can create malware that adapts mid-attack to avoid being stopped by security measures.
4. AI in the Security Operations Centre (SOC): Protecting Against AI-Driven Threats
4. AI in the Security Operations Centre (SOC): Protecting Against AI-Driven Threats
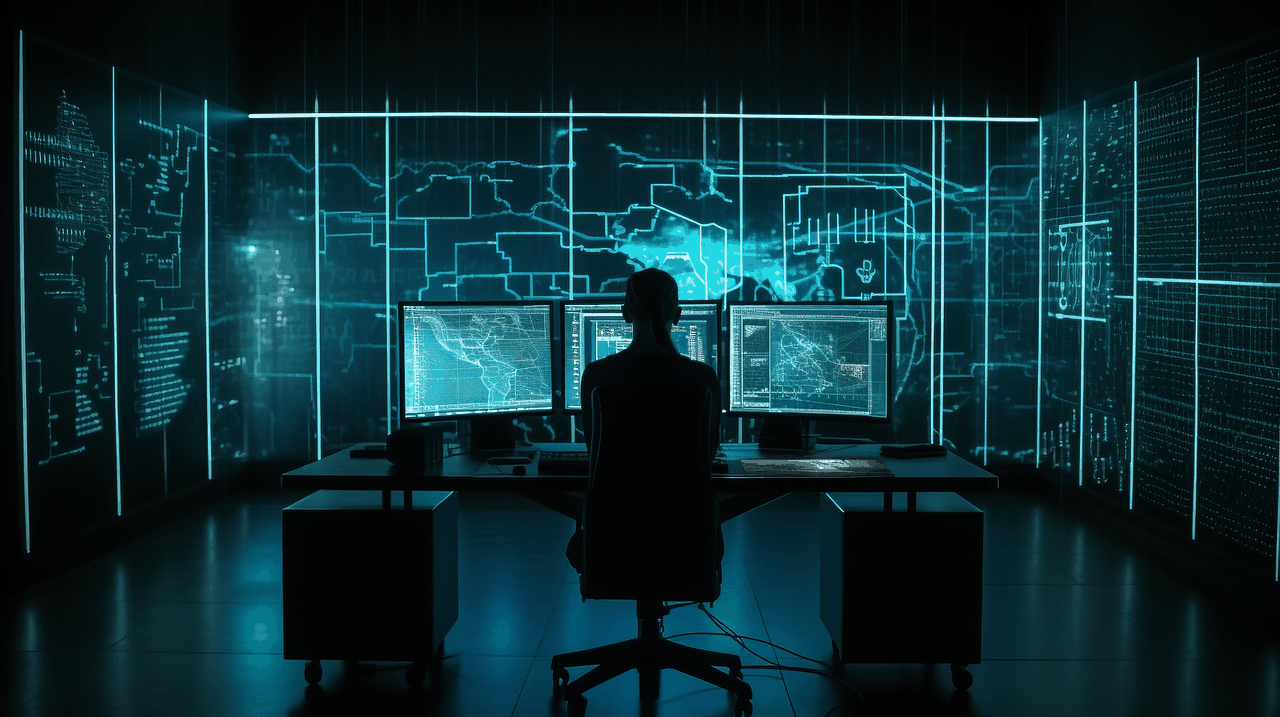
Using AI in Security Operations Centres (SOCs) has transformed how companies monitor and respond to security incidents. One client implemented an AI-enhanced SOC and was amazed by its ability to detect anomalies they would have otherwise missed, and combined with SOAR analysts are able to do more fruitful detection engineering. However, AI in the SOC isn’t without its vulnerabilities, such as data integrity attacks where compromised data can influence SOC outputs.
AI-powered SOCs can detect early indicators of compromise and identify suspicious patterns.
- AI-Powered Network Anomaly Detection: Darktrace offers AI-based systems that analyze network traffic patterns in real-time to identify unusual behaviors that may indicate a breach or ongoing attack.
- Automated Threat Hunting with AI: CrowdStrike provides AI-driven threat hunting tools that continuously scan an organization's environment for indicators of compromise.
- AI-Enhanced SIEM: Splunk's advanced SIEM solution incorporates AI to correlate and analyze log data from multiple sources, providing more accurate threat detection and reducing false positives, detecting anomalies and supporting analysts to write better contextual searches.
- AI-Driven User and Entity Behavior Analytics (UEBA): Exabeam's UEBA solution leverages AI to establish normal behavior patterns for users and entities within an organization, helping to detect insider threats, compromised accounts, or lateral movement by attackers.
AI-Specific Threats: Understanding Key Risks
AI-Specific Threats: Understanding Key Risks
The OWASP Foundation has created a list of the top security concerns for AI-driven applications, which can serve as a roadmap for identifying where AI systems might be vulnerable. Think of this list as a collection of the most common “watch-out” points in AI security. Each risk highlights a different way that AI applications — especially public or in-house models — can be exploited or mishandled if not managed carefully. Here’s a look at these risks and how they apply to today’s AI-driven cyber security landscape.
The table below summarises the OWASP LLM-specific threats and maps them to the relevant sub-topics discussed above, offering a snapshot of where businesses are most vulnerable and which areas warrant careful attention. Refer to genai.owasp.org for further insights. There is an ML equivalent version which has many overlaps with the LLM Top 10. In the below list, it's arguable that an organisations cyber security team (which the SOC could be one part of) should be defending against all threats.
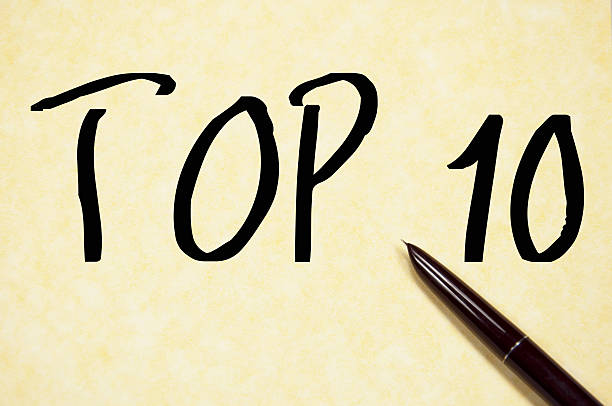
OWASP Top 10 LLM Threat Summary (Version 1.1)
OWASP Security Risk | Description | Relevant Sub-Topics |
---|---|---|
1. Prompt Injection | Crafting malicious inputs to manipulate LLM outputs, often leading to unauthorized actions or information leakage. | Malicious Actors, Public AI Models, In-House AI |
2. Insecure Output Handling | Failing to properly handle or filter LLM outputs, which can lead to unintended disclosure or misuse of generated content. | Public AI Models, In-House AI, Malicious Actors |
3. Training Data Poisoning | Manipulating the model’s training data to influence or corrupt future outputs, potentially leading to harmful or biased results. | In-House AI, Malicious Actors |
4. Model Denial of Service | Attacks designed to overload or disrupt an LLM's availability, impacting its effectiveness in production environments. | In-House AI, SOC Defence |
5. Supply Chain Vulnerabilities | Risks from using third-party models, plugins, or data sources that could be compromised. | Public AI Models, In-House AI |
6. Sensitive Information Disclosure | Risks of unintentionally exposing confidential data in responses due to lack of filtering or model limitations. | Public AI Models, In-House AI, SOC Defence, Malicious Actors |
7. Insecure Plugin Design | Vulnerabilities arising from plugins that interface with LLMs without secure validation or access controls. | In-House AI, Malicious Actors |
8. Excessive Agency | Allowing the model too much autonomy, which may lead to unintended or risky actions without adequate oversight. | In-House AI, Public AI Models |
9. Overreliance | Depending too heavily on LLMs for critical tasks, which could lead to errors or security risks if the model is compromised. | Public AI Models, In-House AI |
10. Model Theft | Unauthorized access to or copying of proprietary models, leading to intellectual property loss and security risks. | In-House AI, Malicious Actors |
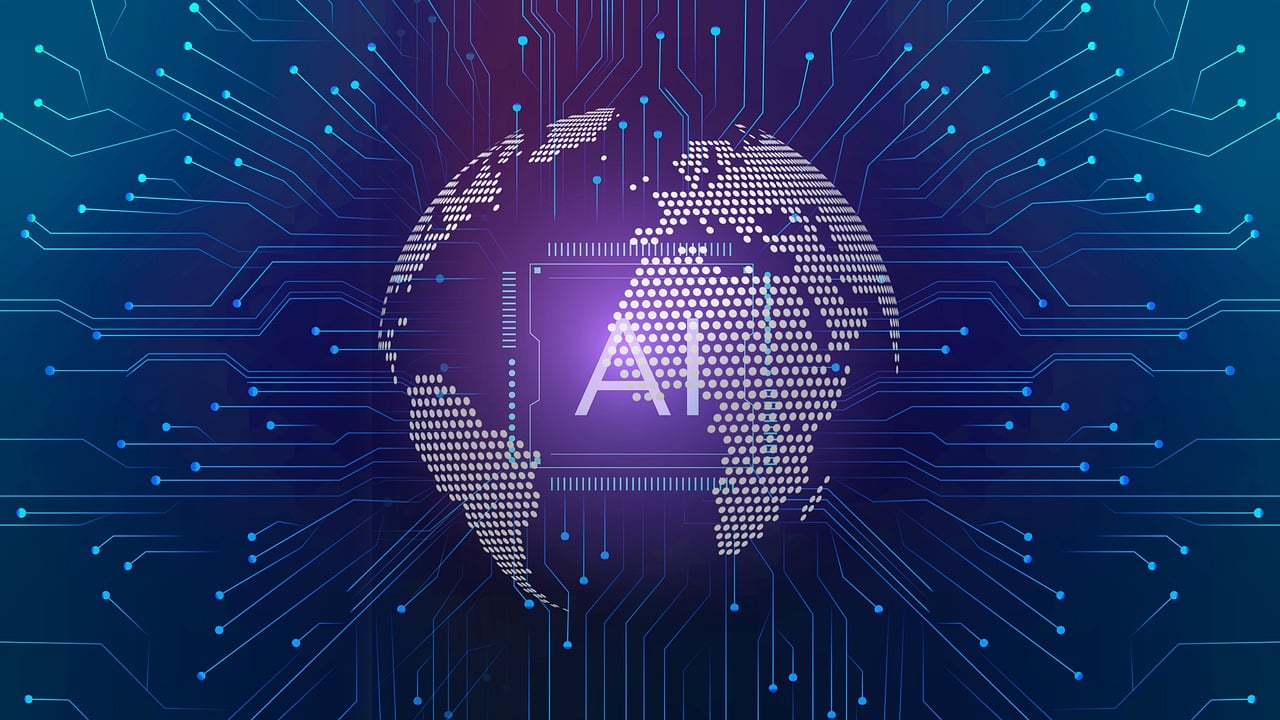